For the second time this March, I was back in London. Spring was in the air, and so was a new kind of pressure. This time, it wasn’t about sustainability. The visit was for The Economist’s Business Innovation Summit. It was a gathering not of futurists, but of business leaders trying to get real about AI. The brief was clear: From AI to ROI. No cheerleaders, or Silicon Valley spin. Just what’s working, what’s stuck, and what’s about to break.
No one here needed convincing that AI is a big deal. The question wasn’t if, it was how. What does real change look like when you’ve got quarterly targets to hit, legacy systems that creak, and a workforce still learning what a token limit is?
Most companies say AI is transforming work. What they’re actually doing is plugging it in like a smart add-on and hoping it sticks. But the ones seeing real change are rethinking everything, not just tweaking at the edges.
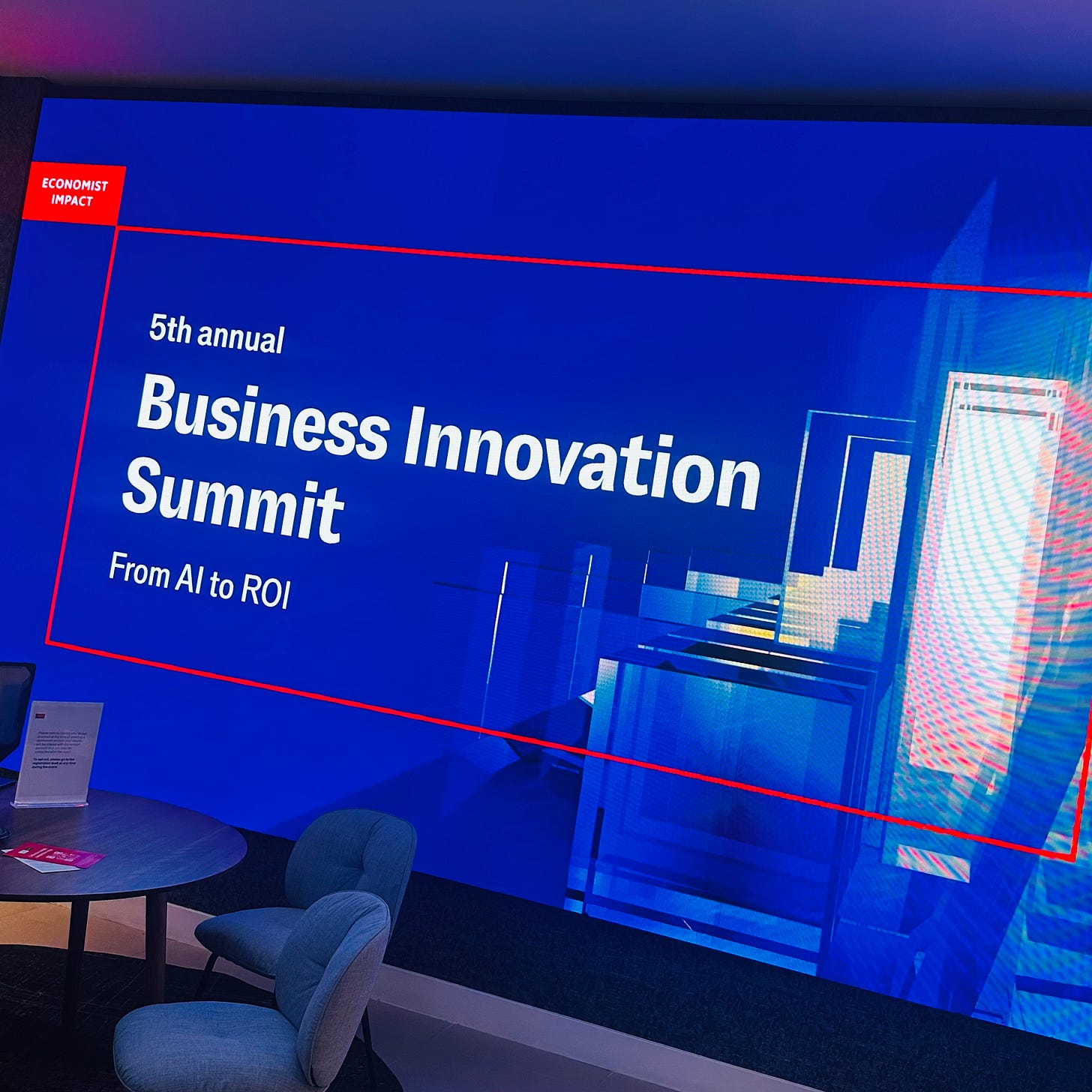
The room wasn’t full of AI dreamers. It was full of people who’ve hit the wall and come back with better questions. Shadow IT. Talent gaps. Energy bills. Pilots that never scaled. Cultural resistance. Burnout. The kind of practical pressure that doesn’t show up in keynote slides, but does show up in budgets and broken dashboards.
The loudest voices weren’t futurists. They were operators. Rebuilding the messy middle: training people before the tech outruns them; swapping automation checklists for real agent workflows; fixing the data mess before dreaming up use cases, and stress-testing risk in a cloud setup that still breaks under load.
And while no one said it outright, the subtext was clear: AI isn’t just about productivity. It’s about power. Who controls it. Who understands it. Who adapts first.
This special dispatch is a snapshot of that moment: the turning point where the AI conversation shifted from promise to pressure. What follows in the next 7,000 words is what I saw, heard, and learned. No hype. Just the reality of what happens after you say “we’re doing AI.”
You can use the table of contents on the left to jump between topics (if you’re reading online), and if you download the Substack app, you can listen to a narrated version of this feature.Let’s do this! 👇🏻
Millennial Masters is brought to you by Jolt ⚡️ The UK’s top web hosting service
1. AI hype is cheap. Strategy isn’t.
Lots of companies are still chasing shiny tools. The serious ones are chasing results. That means starting with strategy and a team that knows what problem they’re solving, not just what tool they’re using.
“Business strategy comes first and foremost,” said Loretta Franks, chief data and analytics officer at Kellanova (formerly Kellogg’s). “It’s foundational to finding the right use cases and the application. [...] We don’t want to be drowning in MVPs that look great on a PowerPoint but can’t scale.”
The pressure is everywhere: to move fast, look busy, and not fall behind. But jumping in without a clear purpose? That’s how you burn budget and stall progress. So the most effective leaders are slowing the rush. Making space to learn. To test. To get people aligned before the tech takes over the agenda.
At Kellanova, that meant launching “curiosity clinics” company-wide, optional sessions on AI. “Our whole executive group has been attending them, sponsoring them, and educating themselves around AI,” said Franks. “They’ve been quite vulnerable in front of broad groups… it’s grounded everyone to the same level.”
That shift in culture, from top-down certainty to something messier, more experimental, came up again and again. “There’s a lot of pressure to be a leader, to have all the answers,” said Jon Lexa, president of Sana. “But the moment you can break down that shield and say, ‘I’m actually just figuring this out as well,’ it can be extremely motivating.”
But no, this doesn’t mean everyone needs to become a coder. “There is a thorough delusion that the C-suite will learn absolutely everything,” said Marcin Detyniecki, group chief data scientist at AXA. “It’s not in a one-week training that you’re going to make a data scientist.”
What matters is knowing where AI fits, what it can unlock, where it breaks things, and what’s likely to slow you down. At AXA, that means applying AI “where it makes sense for your job” and designing training without technical exercises, in partnership with business schools.
Scaling AI means making it fit your world, not pasting in someone else’s use case. “We’re actually not using [our AI] the way our customers use it,” said Lexa. “We’re taking the technology and applying it to our context… that’s what delivers impact.”
The same thinking applies to people. You can’t lift and shift someone else’s plan for that either. “You need to give them the tools to come with you,” said Franks. At Kellanova, that meant investing in soft skill development like storytelling, critical thinking, business relationship management as part of a company-wide “year of development.”
Nadine Thomson, former president at Choreograph, summed up the stakes: “I’ve seen a lot of companies spending substantial amounts on AI and not seeing returns. Unless you’ve got a really defined use case… don’t spend £3 million on it.” Her message? Start small. Stay strategic. And don’t let the hype do your thinking for you.
Recognising that AI is important is easy. Deciding what to do with it (fast!) is where the real tension kicks in.
2. The AI anxiety: Act fast, think slow
Everyone feels the heat to move fast. But speed without structure is risky. The smart play now? Slow down just enough to get it right.
“I think some of us are feeling that fear right now about how things will play out based on the choices we make about AI today,” said Lorraine Barnes, UK Gen AI lead at Deloitte. “Change moves at the pace of business, not the pace of technology.”
That tension cuts through every boardroom: the promise of AI is clear, but putting it to work is another story. “Organisations have systems and controls and processes and infrastructure that, for good reason, will slow the pace at which we can adopt this technology,” Barnes said. “We need to give it space, give it oxygen, allow for the creative process to happen.”
Forget the headlines. Most companies are still figuring it out: testing, tweaking, hoping something sticks. “It’s important to distinguish between Gen AI and AI,” Barnes said. “With Gen AI, we don’t know yet.” Financial firms have had a head start with structured data, legacy systems, and years of modelling. But now, every sector is pushing forward. “We’re seeing fantastically applied use cases in life sciences, around research data, in public sector, in the technology sector… and in lots of cross-cutting applications like legal, customer services.”
Regulation, she said, cuts both ways. “Uncertainty around regulation is likely to hamper the pace of change, but with good regulation, it should help us apply AI safely and appropriately.”
What’s different this time is the very nature of the tech. “It’s very different from other technologies… where we’ve come to expect decision and accuracy. We get through probabilistic models things that appear like creativity, and I think we just don’t know quite how to handle that yet.”
Most execs are already on board. The problem is knowing what that means in practice. “Even the leadership that’s bought in… there is still a big gap in understanding the new technology and the power of the technology and how best to apply it.” She compared today’s frenzy to the early smartphone era. “Here’s the app: what was the question?”
Teams should look beyond small gains and redesign how decisions are made across the business. “It’s quite seductive to get overly focused on the positive benefits of Gen AI,” she said. “There’s a huge upside, no question, but there are also some downsides. We need to take this into consideration.” Her example? A wave of beautifully written AI-generated emails that add zero productivity value.
Yes, the pressure to prove ROI is real. But the bigger risk is doing nothing and falling behind. “I don’t think there’s a risk of jumping in too soon. I think we shouldn’t wait… but making sure that we have the right foundations in place is a no regrets move.”
Employees aren’t waiting for permission. They’re already using public AI tools to get their work done. That’s Shadow IT. “When companies don’t keep pace with my needs as an end user, I’m going out and using publicly available tools to do my job.” That’s not a hypothetical, it’s happening right now. “Our research… tells us explicitly that a majority of employees feel that it will help them in their career progression, it will help them do their jobs.”
Her advice? “Don’t wait. But… give yourselves the time and the patience to deliver the business outcomes and ROI, and really embrace the nuance and the creative process.” Yet it’s not the fear of missing out, but the fear of measuring the wrong thing entirely. Because traditional ROI thinking is already outdated.
3. ROI is broken. AI’s forcing a rethink
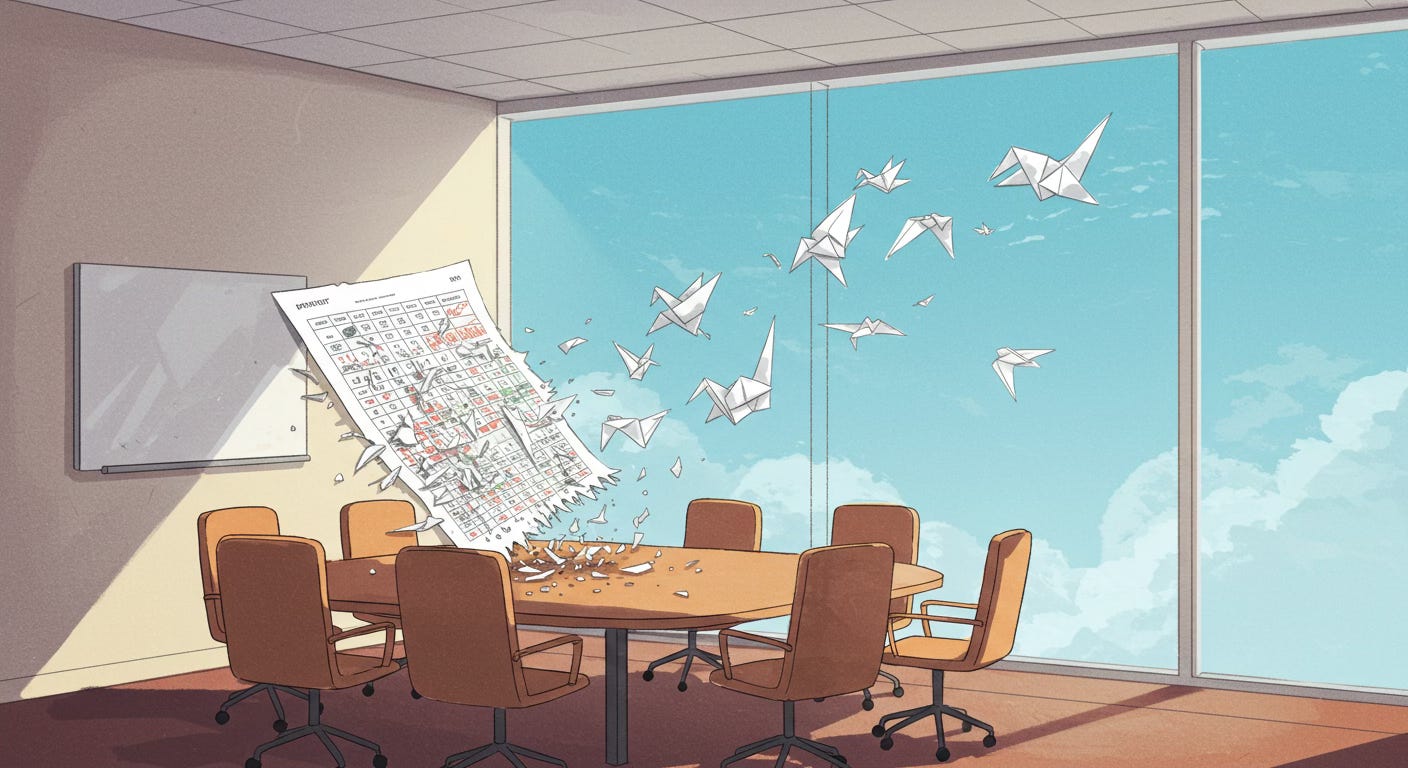
The usual ROI playbook doesn’t work here. AI is surfacing ideas you wouldn’t even know to ask for.
“You’re not going to lose your job to AI. You’re going to lose your job to someone who’s working with AI,” said Costi Perricos, global gen AI lead at Deloitte. “We now have more accountants than ever before, and I think the same thing will happen with software engineering.” This isn’t about jobs disappearing. It’s about rewriting what those jobs actually are, and what skills now matter.
Jessica Hall, chief product officer at Just Eat (takeaway deliveries), put it bluntly: “If you do one of those [request for proposals] today for an AI solution, chances are that during the time you’ve done that RFP, the product has moved on significantly.” Procurement hasn’t caught up with the pace. By the time a process is done, the tech has moved on. Hall’s take? Don’t waste time betting on the best, just start running. “The mindset here has to be around being in the race, rather than choosing the horse.” So she’s hedging her bets, pairing older giants with newer AI players to stay agile.
That pace is forcing companies to invent roles they didn’t plan for. “We’ve been hiring in the UX space, AI specialists to help us level up our customer-facing chatbots and our customer-facing generative AI solutions,” she said. “I know that kind of prompt engineer is a little bit laughed at; is it a real job? But actually, I think being able to write really high-quality prompts… is a genuine skill that everybody needs.”
But the real change isn’t in titles. It’s how we think about work. Aneesh Raman, chief economic opportunity officer at LinkedIn, said the framing itself is flawed. “We’ve done a disservice to the world… by using this term AI literacy, AI skills, AI fluency. That is the equivalent of saying internet skills, internet tools. Like, AI is the big paradigm shift.” Knowing how AI works is one thing. Figuring out how to use it every day, that’s the hard part. “Everyone in this room should be using AI tools; multiple tools for multiple reasons.”
Ikhlaq Sidhu, dean and professor at IE University, cut through the complexity: “The new core competency is speed.” It’s no longer about squeezing margin or cutting heads. Speed and iteration are taking over. “The story that you’re describing here is the singularity story… it is just getting faster and faster.” The real skill now? “Try a new thing… see an opportunity… do this other thing.” It’s about trying fast, failing fast, and moving again. Precision can come later.
Raman’s perspective reframes ROI completely. “We are going to go in this innovation economy, where it’s ease of innovation, not efficiency of production, that’s going to come to the centre.” Soon, we might hear CEOs brag less about cost savings, and more about “a volume of ideas, new products, or features that companies otherwise wouldn’t have been able to do.”
The longer you wait to adapt, the harder it’ll be to catch up. “The change will never be as slow as it is right now,” Raman warned. This is the slowest it’ll ever be again. That’s the new reality.
None of this works without good data. That’s the engine and the limit. “Your generative AI can only be as good as your data strategy,” said Hall. “If I can leave you with one final thought of the ROI, it would be really invest in understanding and developing a top quality data strategy, because that will enable you to get the max out of your AI investments.”
None of this lands unless your people are ready. That means training for action, not just awareness.
4. Skills won’t save you. Mindsets will.
Teaching skills alone won’t cut it. If teams don’t understand how and why to use AI, they’ll miss the point. This is less about learning code and more about shifting culture.
Reskilling sounds good in theory. In practice, it’s disjointed, rushed, and often an afterthought. Until training becomes core to the business, AI won’t make much of a dent.
“If you have managers and leaders that are not AI competent or AI confident, then it’s unlikely that they’re going to have their teams AI-enabled,” said Claire Davenport, chief operating officer at Multiverse.
Resilience doesn’t mean handing everyone a ChatGPT login. It’s helping teams figure out what’s worth doing and how to do it better. “It’s not about being the best in the latest on AI,” said Bastien Parizot, senior vice-president IT & digital at Reckitt (Durex, Finish, AirWick, Dettol and more). “It’s about understanding, ‘let me apply that to our business,’ and who is best to apply that to our business? The people who already know our brands, our products, our research and development.”
Isabell Welpe, professor of strategy at the Technical University of Munich, argued the real innovation is in how we learn. “If you give everyone a personal tutor, an AI tutor that is tailored to what you know, how you learn, what you’re interested in… the time needed to master certain subjects shortens drastically,” she said.
You can’t train your way out of this. Culture eats training for breakfast if the systems around it don’t change too. “It’s not just about the more usage and the more time saved,” said Davenport. “It’s what that actually then translates into in terms of what people are using AI for.”
Multiverse put this to the test. One group got basic CoPilot training. The other got hands-on coaching. The coached group used AI three times more. And the real impact? “They’re actually using it to solve for harder things,” she said.
Reskilling at scale also demands inclusivity, not just urgency. “We shouldn’t forget about our parents, our grandparents, the ageing society,” said Christina Yan Zhang, chief executive of The Metaverse Institute. “If they didn’t give birth to every single one of us, we wouldn’t exist in the first place, right?” She also flagged that one-third of the global population still doesn’t have internet access, and the digital divide risks deepening further without serious intervention. And we need more women leading this transition, she argued, citing gender bias baked into datasets and algorithms.
Zhang raised a bigger challenge: how humans and machines will co-exist, and who gets left behind. “We’re talking about potentially up to 30 billion humanoid robots living with 10 billion people,” she said, referencing Elon Musk’s long-term predictions. “Maybe we should start to teach our kids and ourselves how to live in harmony with our robotic friends.”
But businesses can’t wait for the education system or governments to fix the pipeline. Resilience starts from within. “The people on our programme are probably in their 30s, 40s,” said Parizot. “That’s because they know the business.”
What actually matters now isn’t fluency in code. It’s flexibility, curiosity, and knowing when to ask better questions. “The top two skills now are flexibility and adaptability,” according to Parizot.
Welpe had a simple message for employers: stop making learning feel like a checkbox. “Many companies still have three training days per year. They might want to consider whether they should abandon that and send a signal to the employee: if you want to learn a business skill that is going to be useful, there is no limit.”
Her advice to HR? “Treat AI agents just the way you would treat other applicants. You’ll interview a few AI agents, maybe you’ll put them on probation. Some, you’ll offer a permanent position.”
The companies that treat AI agents like real team members, with structure, purpose and oversight, will be the ones shaping the next decade. Once teams are fluent, the bigger question is balance. Where should the machines take over and where do humans still make the call? That’s where work itself starts to evolve.
But knowing how to use AI is just the start. The next step is putting it to work and making sure the work is worth doing.
5. Don’t replace people. Reinvent work.
AI can remove friction, but only when it’s used with intent. Companies seeing real impact are building systems around people, not the other way round.
The debate isn’t whether AI will reshape work. It already is. The real challenge is using it to boost productivity without gutting what makes teams valuable.
For Danielle D’Lima, vice-president of operations at Depop (social e-commerce), it starts with mindset. “Look for places where AI can create value, rather than just cost savings,” she said. “Outcomes over output.” At Depop, the focus has been on enhancing the user experience, not replacing people, and that principle holds inside the business too. “Let the machine do the heavy lifting and use your humans for things that only humans can do, like creativity, empathy, critical thinking.”
That balance is already being put into practice on the shop floor. Ahmed Khedr, VP of retail digital at e&, described how their telecom stores in the UAE use AI screens and facial recognition to tailor service. “Instead of the agent spending lots of time searching manually… the AI-powered applications already give personalised recommendations,” he said. It’s freed up teams to focus on complex issues and human connection. The result? “Productivity increased by 18%, conversion rate increased by 32%, and the customer experience… above 90.”
The gains are real, but automation still needs a human filter. “Some organisations had to remove [AI-powered customer service] for a specific type of customer,” Khedr warned. “Instead of being promoters, they are not happy with the algorithm.” Human oversight, he stressed, is still essential, especially in customer-facing roles.
Bilal Gokpinar, professor at UCL School of Management, echoed this. “One fascinating finding we have is that… with automation, there is the risk of reduced innovation.” Over-relying on algorithms can dull critical thinking. “If your human workers are not engaged with the technology, then after some point… you’re not going to be able to leverage the entire human capital that you have.”
Still, the fears around mass job losses may be overblown. “61% [of jobs]… would see a redesign,” said Martin Thelle, senior partner at Implement Economics. “Only around 7%… could, over a period of 10 to 15 years, risk being replaced.” And even in automation-heavy roles like customer service, the shift isn’t always negative. “We see not only higher productivity, but also… greater job satisfaction” when AI augments, not replaces, workers, Gokpinar added.
What separates the smart operators from the rest? Start with a strategy, said Peter Jackson, global head of data office (interim) at Schroders. “We wrap this into a data literacy programme… because data is becoming more and more prevalent in people’s jobs.” Training isn’t just for the tech teams. Every role now touches data.
And companies can’t do it alone. “Society has an ethical responsibility to upskill and retrain people,” Jackson said. Universities are stepping up too. UCL is launching hybrid courses that blend marketing, data science, and resource centres to help SMEs build AI capabilities.
Every business will take a different route, but some basics are non-negotiable. “Start with the end in mind,” said Khedr. “Not by the technology, by the problem.” The tech should follow the problem, not dictate it.
The winners won’t be the ones with the shiniest dashboards. They’ll be the ones who design work around people, not platforms.
6. Your new co-worker is an (AI) agent
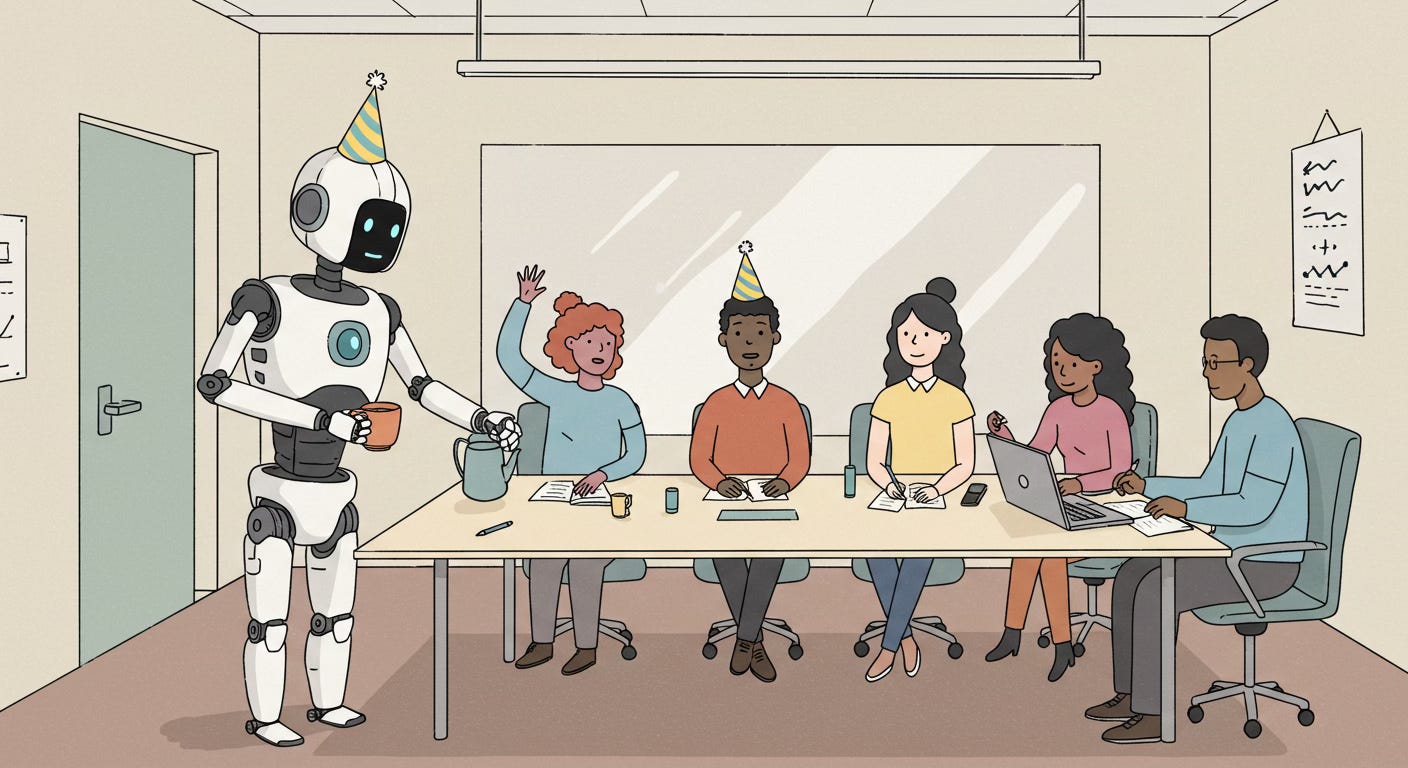
AI agents are no longer just chatbots. They’re planning, deciding, and getting embedded into core workflows, like digital colleagues with real jobs to do.
These agents don’t just respond, they act. They pull data, trigger actions, and even hand off tasks across teams. The impact depends on how it’s done: smoother operations or total upheaval.
“It’s kind of moving over into this world where, as you sort of quite generally [put it], the AI is able to act,” said Yemi Olagbaiye, client solutions director at Softwire. “So we’ve got the understanding bit like as the front end… and we’re now moving over to this world where the AI can act and make decisions on your behalf or on its own.”
“If you look at the retail world, you’ve got companies like Sephora that are having these AI agents… able to almost do like a sort of customer service, upselling, cross selling type of thing,” said Olagbaiye. “In the sports world as well… [the NFL is] giving the ability to analyse the behaviours of sports players… and in real time, give them feedback.”
But the real risk isn’t rogue AI. It’s the system doing exactly what it’s told without questioning the logic. “I don’t think that the risks that we have with these things is Terminator-style like AI gone rogue,” he said. “I actually think that the biggest risk… is more just that the agentic AI does exactly what we tell it to do.”
His analogy? Parenting. “You have your child, and your child grows with these new capabilities… all of a sudden you can walk, you can crawl, talk… With those new capabilities, that’s exciting… but with that comes new risks.”
The solution, he argued, is proper governance from the outset. “It’s about giving someone the specific ownership of that role,” he said. “That might be the Chief AI Officer. It might be the business unit leader… rather than governance being this kind of checkbox thing.”
Organisations also need to get their infrastructure in order. “There are so many things coming here… there’s the sort of data… it’s also about that continuous governing process,” he said. “What happens if there is some hidden bias… and this is leaving these autonomous agents to go off and do a bunch of stuff on their own?”
But this isn’t just a tech upgrade, it’s a whole new way of thinking. “You hire an AI agent for your organisation. You give it not only the data, but also the policies and the context,” he said. “That feels really exciting.” But the real challenge is what happens next. “You don’t want to wait until some sort of scandal happens,” he warned. “Do everything… by design from the beginning.”
What happens when you build your business around agents, not people? Jon Lexa, president of Sana, sees it as a rewrite of how businesses actually run. Forget clunky dashboards and endless admin. With agentic AI, the goal is simple: automate the boring stuff so people can focus on what really matters.
“When we say we’re building agentic AI,” said Lexa, “it’s taking a large language model, combining that with knowledge — that knowledge could be a policy document, contract in a database — and then combining that with instructions and tool use.” These agents don’t wait for prompts; they carry out tasks, trigger actions, and deliver results. “An example could be, I want to analyse the top 10 opportunities in Salesforce from last week. If I write that to an agent, it will identify that it has to select Salesforce, see which opportunities I have access to, and then return those results in a synthesised manner.”
This shift sounds small but it adds up. “You no longer have to funnel around with these horrendous enterprise user interfaces,” said Lexa. “That might seem simplistic, but if you start to compound these small improvements over time, agents can actually unlock quite a lot of productivity for you.”
The real magic comes when those agents start collaborating across systems. Sales teams managing RFPs are a perfect use case. “These requests can have hundreds and hundreds of questions… but your company might have a corpus of old requests,” Lexa explained. “Now, within the agent, you can instruct it to go look at the historical ones, find the ones that are most relevant… and use the historical answers to populate the new RFP, saving you weeks, if not, at least hours of time.”
Lexa didn’t sugar-coat it. “It is not a silver bullet. It’s not going to cure everything. Hopefully not, because I think there’s a lot of purpose to what we do as humans.” There are still limitations. “That’s not something we can solve today,” he said, referring to AI struggles with CAD files and complex design workflows.
And how do businesses do it right? First: strategy. “You’re not going to get away with just having a slide in your quarterly board deck saying that you’re buying Copilot licences for everyone,” Lexa said. It needs to be tailored to your organisation. “Why is it that you think you can optimise your supply chain using agents, but not perhaps legal workflows?”
On the future of work, Lexa didn’t hold back. “Jobs will fundamentally change… don’t try to figure out with your existing workforce where you can kind of plug in AI over time.”
This goes beyond efficiency. It could determine who thrives and who falls behind. For leaders still hesitating, Lexa had a clear message. “We’re at a critical juncture… we’re going to see quite a significant change in how we work, in how we organise ourselves at work.”
AI might not replace your team, but teams that use it well could replace you.
7. On the edge: AI left the cloud
Edge AI isn’t a future trend. It’s already shifting how major industries operate, from aerospace to energy. Whether it’s simulating aircraft or managing home energy use, the action is moving away from the cloud and closer to the front lines. But the transition isn’t seamless. Technical limits and organisational inertia are slowing it down.
“If we are combining the generative AI in the simulation, we sometimes can reduce even 100 times the computational time,” said Grzegorz (Greg) Ombach, head of disruptive research and technology and senior vice-president at Airbus. “It gives us ability to speed up development, increasing the loops of the simulations… we can get better product at the end of it, and it is already applied today.”
This isn’t just about speed. It’s forcing companies to rethink their entire model. Giorgia Molajoni, chief technology officer at Plenitude (gas and electricity sales), explained how edge AI is reshaping the energy sector. “A smart meter is not only a data collector anymore. It becomes an intelligent smart device that can promote action, sometimes even take action… the same person can choose to be a consumer, a producer, so prosumer, or even somebody that just stores energy.”
That change flips the traditional energy model, handing more control to individuals. “What used to be strongly calculated by the company providing the energy now has to match demand and response and storage offer from a multitude of people. That changed your business model from a centralised one to distributed one, which changed completely the parity of the energy business.”
Still, tech doesn’t fix everything. “Applying AI everywhere doesn’t make sense in many cases,” said Ombach. “There are use cases which we have seen that applying AI costs you more than not applying it, also from an energy cost perspective.” In safety-critical areas like aviation autopilot systems, for instance, Airbus avoids AI altogether due to certification challenges. Instead, they focus it where the benefits are clear, like improving satellite imaging resolution or boosting internal productivity through knowledge management tools.
In other sectors like retail and finance, scaling hits both tech and team barriers. “Make sure you have a workflow that is educated on things like using AI,” said Tahmid Quddus Islam, vice-president of innovation and technology at Citi. “Great technologies come out and everyone’s super excited… but they might not necessarily know how to use it to the best of their ability.”
Ruth Miller, principal consultant at Lenovo, agreed. “Get a business case underlined and agreed with the business. Not just from a POC, but from a pilot and a full production rollout. Because I see a lot of things falling in between.” Inside global firms, tangled structures often kill momentum before it starts. “There’s about 25 different companies within that retail group. Another five which are only partially owned… how do you tell all those different countries, different managements, that they’re going to roll out something they see no value in?”
The real wins go to teams who can tie AI to hard numbers that make sense in the boardroom. “This saves you money, this creates you value and make it a big number, so everybody on the board understands,” said Miller.
Ombach sees the edge as a launchpad, where AI and robotics are already starting to converge. He expects generative AI and robotics to converge fast, particularly with smaller, more efficient models running directly on edge devices.
Molajoni’s eye is on what happens next, when machines don’t just help humans, but start working with each other too. “I’m interested in how actually human, 100% human, and machine will interact and how we’re going to start to see machines interacting with machines as well. It’s going to be a cultural revolution.”
The ripple effects go beyond business. This shift is already reshaping how we live. “Can you take a phone off a small child now?” said Miller. “They’ve grown up with that. From babies with tablets… technology is part of their lives. It’s a different way of thinking, working and behaving that we’re developing.”
And with all this speed and intelligence comes a price. AI might be smart, but its carbon footprint is getting harder to ignore.
8. AI’s dirty secret: The infrastructure crisis
AI is scaling fast, but so are the power bills. Efficiency gains aren’t keeping up with the demand. 📰 I’ve covered this more in depth in my dispatch from the Economist Impact 10th anniversary Sustainability Week here.
As generative models roll out across more teams, a quieter issue is starting to surface: the infrastructure is buckling. Electricity demand is surging. In some regions, even water supply is becoming a constraint.
“This power story… we’ve known this problem for a long, long time,” said Tikiri Wanduragala, senior consultant for infrastructure solutions at Lenovo. “But what AI has done is put it on steroids.”
AI scaling is now “three times, five times, in some cases, ten times” more demanding. It’s forcing companies to rethink everything from power supply to cooling systems. “Infrastructure — very boring. No one paid any attention,” Wanduragala said. “Suddenly, in the last 36 months, it’s a huge interest.”
The impact is real. Microsoft’s emissions have risen by a third. Google’s are up by half. Even the most efficient cloud giants are starting to strain. “We’ve eaten up a lot of that [efficiency] going forward,” Wanduragala said. “Customers are talking more about hybrid AI… bringing stuff in house.”
It’s also widening the energy performance gap between organisations that track impact and those that don’t. “Do you have accurate data about how your infrastructure is operating, how your workloads are running?” he asked. “This gives you these little secret pieces of information.”
Cooling remains a huge hidden cost. “Forty percent of new energy is used for cooling,” he said. “And water… for some data centres [is] being difficult to deploy because of restrictions.”
But the same tech causing the spike could also help cut it. Lenovo is already testing AI to cut waste in packaging and supply chains. “There’s a lot of data, a lot of efficiencies that are possible,” he said.
His advice to companies: don’t wait for regulation to force your hand. “Even if you don’t care about profitability, even if you don’t care about carbon,” he said, “you should still be thinking about this story.” Because AI is only going to get smarter and hungrier.
9. AI is a new secret ingredient
AI isn’t a side project at Deliveroo (takeaway and grocery deliveries). It’s now baked into how the business grows and makes decisions. For CTO Dan Winn, it only counts if it changes something real. “We’re laser focused on what customers want and solving the needs of our consumers, our partners and our riders,” he said.
Take customer feedback. Like most companies, Deliveroo uses NPS surveys to gauge satisfaction. But there’s a flaw: only the very happy or the very angry tend to respond. “It doesn’t represent the majority of interactions you’re having with customers,” said Winn. So the team built a generative AI system to analyse the full scope of customer support chats and generate a synthetic NPS score, one that’s far more representative of real sentiment. “We tested it… and tuned it to a place where it is very representative of what a customer would say if they answered the survey.” The result? A faster, truer read on how customers actually feel, and sharper product calls as a result.
AI’s also reshaping the back end, especially in logistics. For a company delivering everything from a burrito to a week’s worth of groceries, assigning the right number of riders per order is critical. “Historically, we had to guess based on the cost or the number of items,” said Winn. But that left too much margin for error. Now, they use AI to scan item images and descriptions, estimate weight and volume, and assign the right number of riders. “It drives cost efficiency… If two riders go out, that cost is entirely borne by us.”
Beyond operations, Winn sees AI playing a defining role in how software gets built, and who builds it. “We’re seeing an evolution in our engineering teams,” he said. “Increasingly, engineers will operate as tech leads, directing the work of a small fleet of software engineering agents.” He sees that shift speeding things up and giving engineers more room to think. “For engineers that embrace it, it’s a superpower.”
Winn also knows AI’s not just about speed. It ties back to the business model. “We’re now a profitable company, producing positive free cash flow,” said Winn. “That enables us to focus more entirely on serving the needs of customers and partners.”
AI is making conversations cheaper and smarter. Deliveroo’s betting that soon, most chats with customers will start with an agent. “There’s a sea change coming in the next two years,” Winn predicted.
But none of this works if the basics break. And the cloud is starting to creak.
10. Cloud chaos is coming
In today’s cloud-heavy world, outages aren’t rare. They’re inevitable. So how prepared are businesses to deal with the next big failure?
That was the warning from Mark Gradwell, board member at Zero Outage Industry Standard: “Go back and make sure that you have done a risk assessment recently. Things change all the time. Technology changes all the time.”
Multi-cloud setups are trending, but they’re no magic bullet for staying online. “There are definitely benefits… but I would argue the only way that you will have resilience is by adopting a multi-cloud strategy — especially if you’re working in a multinational organisation.” The catch? “There is definitely complexity that you need to consider in the management.”
More companies now want options: independence from vendors, control over where their data lives, and flexibility across borders. “If you’re a multinational doing business across the globe, being able to select cloud providers in Asia or in Latin America or in the US or in Europe becomes super important.”
It’s not just about picking the right tech. Companies need to practice failure like it’s part of the job. “I cannot stress this enough, how important running tests are and making sure that you do them on a regular basis.”
That starts with knowing your numbers: how fast you can recover, how much data you can lose, and which systems your teams are quietly leaning on. “Some outages are caused through carelessness… but when we’re under pressure, sometimes we don’t make the best decisions.”
In one case, a CEO dismissed an outage warning during a client visit, assuming it was a joke. “He phoned in… and was told the service could be restored in a few minutes. It didn’t take an hour — it took about two hours,” Gradwell said. “We were overly reliant on the hyperscaler.”
The failure? “Humans do funny things under pressure,” he added. “Testing and making sure your business continuity plan isn’t just gathering dust — that’s key.” What matters most? Communication. Who hears what, when, and how, all of it needs planning. “Knowing how the communication is going to go out, the frequency of the communications, who the stakeholders are, is absolutely key.”
Tech fragility is just one pressure point. The other is policy, and right now, the rules are still being written.
11. Regulators are winging it
AI is having its policy moment. Governments are racing to write the rules, weighing risk frameworks against economic ambition. But if businesses want rules that work, they need to speak up — now.
“The AI Act was very much drafted with that kind of mindset,” said Jon Steinberg, Director of Global Policy Campaigns at Google, referring to the EU’s risk-based approach to AI regulation. “And then Gemini, ChatGPT happened… there was a shift.”
That shift sparked a wave of public debate and political urgency, with interventions from tech leaders, academics and governments. “We had the Bletchley Park summit… the follow-up summit in Seoul… very much focused on safety and risks,” said Steinberg. “Where I think we are now… is a pendulum swing back towards the opportunity.”
For Google, that swing is long overdue. Steinberg quoted CEO Sundar Pichai: “AI is too important not to regulate, but too important not to regulate well.” Countries like the UK, Japan and Singapore are pushing for pro-growth policies over blanket restrictions. “That shift… we think is really positive.”
Still, Steinberg said that Google remains in favour of regulation, as long as it sticks to risk-based logic. “We’ve been supportive of [the AI Act’s] fundamental framework… we were very active participants in that debate in a constructive way,” he said. But as the legislation evolved, concerns emerged.
“In the final stages… they introduced a separate category of rules around what they call GPAI — general purpose AI,” he explained. “They basically said… these models are so big that they need their own class of rules because they are inherently risky. We disagree.”
Google’s concern? New categories like GPAI could slow down innovation before it even starts. “That’s when you run the risk of closing your market off from the innovation, the growth and the opportunity that it costs,” said Steinberg. And other countries are watching. “Governments are thinking twice before copying and pasting.”
That concern is particularly acute when it comes to transparency. “There is some merit to having transparency about how models are built,” said Steinberg. “What we are mindful [of] is when those obligations potentially threaten trade secrets [and] competitive advantage.”
What about the role of public opinion? “Governments are representing the interests of their citizens,” Steinberg said. “But we also want to be mindful… that we don’t regulate a hype cycle.”
He shared a cautionary tale from Google’s early work on self-driving cars. “I can very clearly remember going to a meeting with an unnamed transport ministry where they said, ‘We’re going to write a law about how self-driving car technology should be governed’… before the technology was proven.” The lesson? Don’t legislate the unknown.
As the rules shift, some fear a global race to relax standards. Steinberg doesn’t think so, but admits there’s competitive tension. “Perhaps some friendly… competition about where is the best place to develop and deploy.”
Europe might be leading on AI rules, but that lead is starting to sting. “There’s a reason why companies like Google, like OpenAI, like Meta… are choosing to launch products in Europe later,” Steinberg noted. “That’s the risk.”
Because whether the policy’s perfect or not, the market’s already moving. The front-runners aren’t patching AI onto old systems. They’re rebuilding from the core. Policy matters, but no amount of lobbying can make up for a weak foundation. The real question is how your business is built.
12. AI isn’t a feature: Build for what’s next
AI isn’t on the sidelines anymore. It’s centre stage, and the stakes just changed. The hesitation’s gone. Now it’s about pace, clarity, and consequence.
What I heard at the Business Innovation Summit was clear: the age of AI pilots and “experimentation” is over. If you’re still exploring use cases, you’re already behind. The best companies aren’t just deploying AI. They’re rebuilding around it.
This isn’t about doing more with less. It’s about doing things you couldn’t do before. The real shift isn’t technical. It’s cultural. AI changes how an organisation senses, decides and moves. That demands rewiring, not tweaking.
Success won’t hinge on data or model size. The edge now is speed of adaptation. As one speaker put it: “There are no technical problems. Only people problems.” The boldest companies are solving for that first, redesigning how work gets done, not just which tools sit in the stack.
But many are still using AI to speed up old processes. Faster forecasting. Cheaper customer service. Smarter pricing. That’s not transformation. That’s efficiency theatre.
The real gains come from automating judgement, not just tasks. From surfacing insights that no team would have known to look for. From rethinking how decisions happen.
Most companies are layering AI onto broken systems. A few are rebuilding the machine. Those are the ones reshaping the market. The rest are stuck trying to optimise failure.
The next stage isn’t about scale. It’s about alignment. Leaders who can connect culture, infrastructure, and product will move faster than regulation and faster than competitors. That window is already closing.
This shift won’t be reversed. The technology has changed, and so has the pace. What happens next depends on how well your business listens, learns, and moves. There’s no safety net for the slow. But there’s no ceiling for the fast either.
Don’t miss my Booksmart primer: AI, power & the future: What the smartest people are actually saying about AI — without hallucinations.
What GPT won’t say about real AI power 🦾
If you’re a builder, leader, or investor, the next decade will be defined by whether you understand the system you’re operating in, and whether you choose to know the system or be ruled by it without realising.